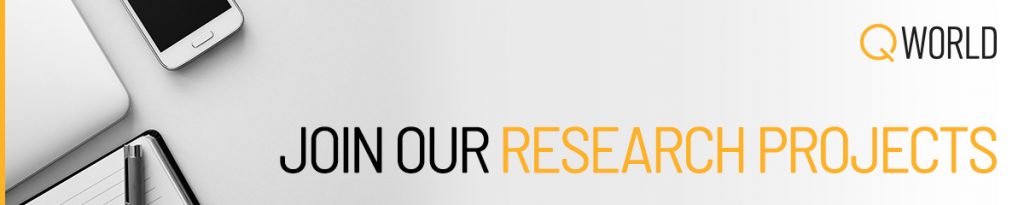
QResearch promotes and helps in making open-source and high-level quantum science. We believe that high-quality research requires a simple reconstruction of results and easy access to the results by anyone. Finally, sharing our experiences is important – the more people with different skills join, the more likely it is that the research outcomes will meet high-quality criteria.
QResearch offers help to researchers in the following ways:
- We help to find participants for the project to help in doing science;
- We encourage and help research projects to keep open-source standards, which includes the ability to reproduce their results;
- We advertise the research outcomes on our social media.
Research Project Proposal Form for Mentors >>
Each research project team has to follow the rules of Research projects.
Contact: qresearch [at] qworld.net
Past Research projects
RP-002
Mentor(s): Aurthur Vimalachandran Thomas Jayachandran
Intern(s): Mehmet Ege Bozdemir, Engin Bahri Baç, Shrey Biswas, Amaury de Miguel, Marija Šćekić, Celestine Ikoki
Project description: The overall goal of this MSCA is to develop a set of algorithms that would allow for seamless decisions to solve the problem of multi-period portfolio optimization containing multiple crypto assets, to maximize the Sharpe ratio using quantum machine learning along with quantum natural language processing for understanding market positioning. The results will be benchmarked with existing crypto ETFs (exchange traded funds).
RP-004
Mentor(s): Özlem Salehi
Intern(s): Ashish Kumar Singh Arya, Fabiola Cañete Leyva, Dhruvi Kapadia, Ludmila Augusta Soares Botelho
Project description: Subproject 1: D-Wave Systems platform allows the creation of Markov Random Fields and provides with a way to sample for solutions. In this work, we are applying D-Wave Markov Random fields to the tasks of melody completion and harmonization. Subproject 2: Music reduction problem is the process that reduces the number of tracks in a multi-instrument music piece so that the resulting music consists of less number of tracks and preserves the characteristics of the original piece as much as possible. In this work, we use quantum annealing to model and solve this process. Subproject 3: Counterpoint is a technique of composing music where multiple rules should be followed. In this work, we use quantum annealing to generate counterpoint music.
RP-006
Mentor(s): Daniel Oi
Intern(s): Tuğba Özbilgin
Project description: The Satellite Quantum Modelling & Analysis (SatQuMA) toolkit is an open source suite for the development of satellite QKD systems. The project will extend its capabilities to incorporate more detailed Space-Earth channel models, entangled dual downlink, and CV-QKD protocols. This will then be used for comparative performance studies of satellite QKD configurations and inform system design.
RP-007
Mentor(s): Jibran Rashid
Intern(s): Shantanu Misra, Vaishnavi Manikrao Markunde,
Project description: Develop introductory tutorial workshop style content on quantum contextuality and nonlocality, leading to small open questions that can be explored computationally.
RP-009
Project name: Logistics Optimization using Quantum Computing
Mentor(s): Paweł Gora (QWorld & University of Warsaw & Quantum AI Foundation)
Members: Turbasu Shaterjee, Carlos Kuhn, Ishmam Shah, Arnav Das, Joshua Keene, Megashrita Das, Walid El-Maouaki, Shantom Borah, Asish Mandoi, Avneesh Verma, Justyna Zawalska, Antonio Jesus Garcia Palomo, Kaustuvi Basu, Shandirai Tunhuma, Sakibul Islam Sazzad, Muhammad Usaid, Rajarsi Pal, Naman Jain, Shivalee RK Shah, Tarun Vikas Vinnakota, Vardaan Sahgal, Artemiy Burov, Katarzyna Nałęcz-Charkiewicz, Priyanshu Pansari, Francisco Del-Rio, Munawar Ali, Marek Wieczorek
Project description: The project’s goal is to investigate how quantum computing may help in optimizing logistics, e.g., solving combinatorial optimization problems like the Vehicle Routing Problem and its variants (e.g., TSP, mTSP, CVRP, CVRPTW). We investigate algorithms and approaches like QAOA, VQE, quantum annealing, graph coarsening, quantum local search, HOBO, quantum neural networks and their variants.
RP-001
Project Name: Implementing QFA algorithms on noisy devices
Leader(s): Dr. Abuzer Yakaryilmaz (QWorld & University of Latvia) and Ozlem Salehi (QWorld & Ozyegin University)
Member(s): Utku Birkan (BS Students in Middle East Technical University), Cem Nurlu (BS Students in Bogazici University), and Viktor Olejar (MS Students in Pavol Jozef Safarik University in Kosice, Faculty of Science)
Description: We are working on implementing two basic quantum finite automaton (QFA) algorithms on currently available quantum computers and analysing the performance of these machines based on the experiment results. We are also using different methods to modify the algorithms in order to get better results against noise and investigating error mitigation techniques to reduce the effects of noise.
This project was initiated during QIntern2020.
Acknowledgements: Abuzer Yakaryilmaz is partially supported by ERDF project Nr. 1.1.1.5/19/A/005 “Quantum computers with constant memory”. Utku Birkan and Cem Nurlu are supported by TÜBİTAK scholarship “2205 – Undergraduate Scholarship Program.
RP-003
Leader(s): Aritra Sarkar
Member(s): Dhanvanth Balakrishnan, Sarang Gosavi, Bao Bach, Soham Prashant Bopardikar, Tamal Acharya, Muhammad Usaid, Kaushal Kishor Gagan
Description: QKSA is a formal framework for resource-optimized universal reinforcement learning. It is the generalization of UAGI models like AIXI and KL-KSA for quantum environments. In this QWorld Research Project we refined some theoretical and implementation aspects of this proposed framework.
RP-005
Leader(s): Adam Glos
Member(s): Mostafa Atallah, Claudia Zendejas-Morales, Vyron Vasileiadis
Description: Recently new space-efficient versions of Quantum Approximate Optimization Algorithm appeared for the Graph Coloring or Traveling Salesperson that uses much less qubits than the standard one. In this project, we will develop software implementation for these new algorithms in Qiskit.
RP-008
Leader(s): Aritra Sarkar
Member(s): Bao Bach, Sarang Gosavi, Tamal Acharya, Akash Kundu
Description: This research is a continuation of the QIntern 2021 project on “Reinforcement Learning Agent for Quantum Foundations”. We studied 3 different aspects of the Quantum Knowledge Seeking Agent: (a) algorithmic information geometry of universal quantum computation, (b) thermodynamics of quantum automata and programs, and, (c) an application framework for quantum accelerated causal tomography.